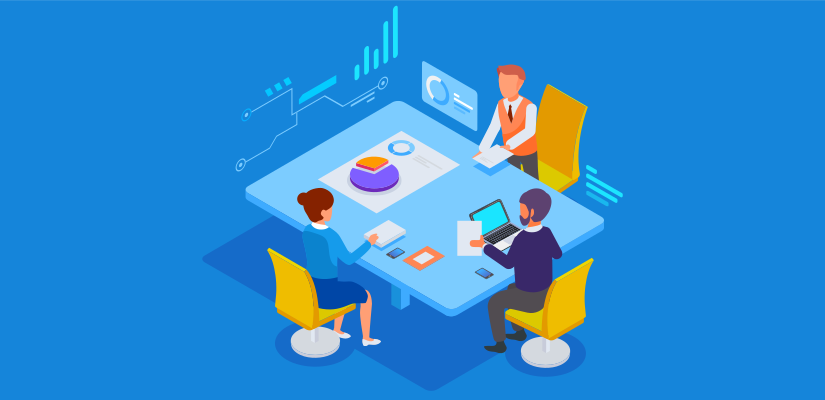
Demystifying Supervised Learning: A Comprehensive Guide
Have you ever wondered how Netflix knows exactly what show to recommend next? Or how your email automatically filters spam? Welcome to the fascinating world of supervised learning – the backbone of many AI applications we interact with daily.
What is Supervised Learning?
Picture yourself teaching a child to distinguish between cats and dogs. You show them different pictures, telling them “This is a cat” and “this is a dog.” Eventually, they learn to identify new animals based on what they’ve learned. This is essentially how supervised learning works – we train machines using labelled examples to make predictions about new, unseen data.
Supervised learning is a fundamental concept in the field of machine learning. It involves training a model on a labelled dataset, which means that each training example is paired with an output label. The goal is for the model to learn the mapping from inputs to outputs so that it can predict the output for new, unseen data. Let’s dive into the details of supervised learning, its types, algorithms, and applications.
Key Components
- Data Collection: Gather a dataset that includes input features and corresponding output labels.
- Data Preprocessing: Clean and preprocess the data to make it suitable for training.
- Model Selection: Choose an appropriate algorithm for the task.
- Training: Train the model on the labelled dataset.
- Evaluation: Evaluate the model’s performance on a separate test dataset.
- Prediction: Use the trained model to make predictions on new data.
The Learning Process
-
Data Preparation
Before any learning can begin, we need to prepare our data:
2. Feature Engineering
Creating meaningful features is crucial:
Types of Supervised Learning
Supervised learning can be broadly categorized into two types:
- Classification: The goal is to predict a discrete label. For example, classifying emails as spam or not spam.
- Regression: The goal is to predict a continuous value. For example, predicting house prices based on features like size, location, etc.
Common Algorithms in Supervised Learning
Several algorithms are commonly used in supervised learning, each with its strengths and weaknesses. Here are a few:
- Linear Regression: Used for regression tasks. It models the relationship between the input features and the output using a linear equation.
- Logistic Regression: Used for binary classification tasks. It models the probability of a binary outcome.
- Decision Trees: Used for both classification and regression tasks. They model decisions and their possible consequences as a tree structure.
- Support Vector Machines (SVM): Used for classification tasks. They find the hyperplane that best separates the classes in the feature space.
- K-Nearest Neighbors (KNN): Used for both classification and regression tasks. It predicts the output based on the k-nearest training examples in the feature space.
- Neural Networks: Used for both classification and regression tasks. They are composed of layers of interconnected nodes that can learn complex patterns in the data.
The Mathematics Behind Supervised Learning
Let’s dive into the mathematical foundation. Don’t worry – I’ll break it down step by step!
Linear Regression Example
For linear regression, we’re trying to find the line that best fits our data points. The equation looks like this:
y = βₒ + β₁x + ε
Where:
- y is the predicted value
- βₒ is the y-intercept
- β₁ is the slope
- x is the input feature
- ε is the error term
To find the best values for βₒ and β₁, we minimize the Mean Squared Error (MSE):
MSE = (1/n) Σ(yᵢ – ŷᵢ)²
Where:
- n is the number of samples
- yᵢ is the actual value
- ŷᵢ is the predicted value
Key Components
- Training Data: A dataset consisting of input features (X) and their corresponding labels/outputs (y)
- Learning Algorithm: The method used to find patterns in the training data
- Model: The mathematical representation learned from the data
- Prediction Function: The ability to use the model to make predictions on new data
Types of Supervised Learning Problems
1. Classification
When your target variable is categorical (e.g., spam/not spam, cat/dog), you’re dealing with a classification problem. Let’s visualize a simple binary classification:
Multi-class Classification
Here’s an implementation of multi-class classification using Random Forest:
2. Regression
When predicting continuous values (e.g., house prices, temperature), you’re working with regression. Here’s a simple linear regression visualization:
Advanced Regression Techniques
Ridge Regression (L2 Regularization)
Lasso Regression (L1 Regularization)
Loss Functions and Optimization
Common Loss Functions
- Mean Squared Error (MSE) for regression:
- Cross-Entropy Loss for classification:
Gradient Descent Implementation
Model Evaluation and Validation
Cross-Validation Implementation
Learning Curves Analysis
Hyperparameter Tuning
Grid Search Implementation
Advanced Topics
Ensemble Methods
Feature Selection Techniques
Conclusion
Supervised learning is a powerful tool in the machine learning toolkit. Whether you’re a beginner just starting or an experienced practitioner, understanding these fundamentals is crucial for building effective machine learning solutions.
Remember: The key to success in supervised learning isn’t just about algorithms and mathematics – it’s about understanding your data, choosing the right approach, and continually iterating to improve your models.
Resources for Further Learning
- Books
- “Introduction to Statistical Learning” by James, Witten, Hastie, and Tibshirani
- “Pattern Recognition and Machine Learning” by Christopher Bishop
- “Deep Learning” by Goodfellow, Bengio, and Courville
- Tools and Libraries
- scikit-learn
- TensorFlow
- PyTorch
- XGBoost